Becoming a Wizard-of-Oz learning assistant
Part of “Letters from the Lab”, a series of informal essays on my research written for patrons. Originally published February 2023; made publicly available January 2024. You can also listen to this essay (17 minutes).
As I described in December, I’m experimenting with some unusual (for me) new research methods. Data analysis and stacks of user interviews have given me a ten-thousand foot view of learning in action. Now I’m taking the opposite approach. I’m diving in close, trying to understand the emotional and practical consequences of individual learning actions and design decisions, over time.
To restate my (admittedly ludicrous) aspiration: I’d like to invent an utterly transformative environment for learning and growth. I want to induce an uncanny, almost alien, feeling of effortlessness and proficiency. So far, I’ve chased that goal by building and iterating on scalable systems. But now, before systematizing or scaling anything else, I aim to produce that uncanny sensation for a single person. I want to make one person feel that they’ve been granted impossible superpowers. Then if I can do it again, and again, I hope I’ll see how to bottle that lightning in a system—a much more powerful system than I could create by iterating “in system space.” If nothing else, I expect this N-of-1 approach will produce some unusual insights along the way.
Meet Alex
So, for the past month, I’ve been acting as a “personal learning assistant / coach” for Alex (name and gender randomized). He’s a creative and driven adult, employed at a startup. Last year, through friends, he met some people working on an obscure problem in physics. Alex became absolutely obsessed. He decided that he had to try to contribute. There was just one “small” problem: he hadn’t studied much advanced physics.
In December, Alex embarked on a six-month quest of full-time study. He aims to understand the state of the art and to become a competent participant in conversations about this problem. He hired two tutors (one for math, one for physics) and began to work through related papers and textbooks.
I suggested that I’d ride alongside his learning process, like a little daemon sitting on his shoulder, and I’d help however we thought might be most useful. Of course, I expected to intervene with memory systems: Alex already had an Anki practice and some experience writing prompts for conceptual material. But I left the scope deliberately open, to make space for generative experiments—whatever seems appropriate to the problem at hand.
It’s an uncomfortable arrangement for me… and that’s good, I think. My practice, my experience, and my culture fixate on abstraction and systematization. Some part of me objects that N-of-1 insights are “fake”, that I’m not “doing real work” when I hack together bespoke one-offs for a single user. It’s been gratifying (and challenging) to confront these preconceptions, to stretch my practice in new directions. Happily, now four weeks into this experiment, I feel more richly connected to the design space in many ways than I have in years.
The force of a live project
As I listened to Alex’s plans and to his tutoring sessions, the first thing I noticed was the constant force exerted by his project—the papers he wants to understand but can’t, the arguments he can’t evaluate.
Here’s an illustrative example: Alex’s tutoring sessions are largely driven by a list of questions he’s brought, not by the tutor prescribing what he should learn next. Alex’s questions aren’t abstract or academic. They’re live blockers for something he cares about immensely. His project drives the learning loop: deciding the next thing to learn, assessing when it’s understood well enough, choosing to move on. And this particular project demands deep understanding at every step.
I’ve interviewed and observed dozens of learners over the past couple years, but this was a new pattern of learning behavior for me. And my instinct is that it provides exactly the sort of pressure my work needs.
The serious participants I’ve previously observed have mostly followed two consistent patterns of behavior.
The first pattern, which I’ll call Syllabus Learning, frames the goal as “learning Subject X.” Sometimes this means trying to pass a test or a job interview. Sometimes it’s a felt sense of obligation or “should”, like “I’ve always felt I ‘should’ learn statistics properly.” Sometimes there’s a project or interest in mind—“it’d probably help my data science work to brush up on probability theory”—but that project isn’t driving the learning loop, day-to-day. The common thread is that the learner’s emotional connection is abstract. An external structure (often a course or a textbook) mostly drives the learning loop: decides what to learn next, when it’s understood well enough, and when to move on. Most of these learners aren’t really trying to carefully internalize the material; they’re trying to “finish the course.” Syllabus Learning tends to be satisfied if the learner can “make it through” the readings and the exercises they’re “supposed to” do.
I’ll call the second pattern Exploration Learning. People reading Quantum Country because they’re “curious about quantum computing.” Sunday reading over coffee—grazing for new knowledge to spark joy or to inspire future action. A sense of novelty and interest drives the learning loop: decides the next thing to learn, when it’s understood enough, choosing to move on. Because that curiosity-directed impulse often doesn’t extend to details, Exploration Learning often aims to internalize results, methods and ideas, but not necessarily the foundations needed to fully explain them.
It may sound like I’m disparaging these patterns of learning. That’s not my intent! These patterns are useful; people (including me) follow them for a reason. Syllabus Learning is a low-cost way to get a basic footing in some topic. Exploration Learning is great for introducing yourself to a wide swath of ideas. In both cases, initial exposure can guide future projects and deeper study.
But speaking now as a designer, I’m trying to create environments which can help people deeply internalize difficult topics. Such an environment would likely help both Syllabus and Exploration Learning. But these patterns don’t really supply the right pressures to help me create that environment. It’s like trying to design a race car by iterating with commuters when they’re driving on city streets. They’re like: “Yeah, it seems like it could go pretty fast.”
So the first big insight I’ve gotten from my work with Alex is: wow, okay, this is the pressure I want. Fiery Learning. He’s driven by a project which demands that he understands difficult material. His emotional connection to the project pushes him to truly understand the material, rather than just “get through it” as in Syllabus Learning. And unlike in Exploration Learning, this project insists on understanding in great detail. This is a demanding pattern of learning. Alex is truly struggling without augmentation. He would very much like help. He’s taking the race car out on a punishing track, trying to beat a formidable time. Any slight changes in performance become extremely salient; any small difficulties become major irritants. It’s an intensely high-signal, high-energy context for me as a designer.
Tutoring transcripts as powerful design inputs
One of the first interventions I proposed was: suppose I give you a special purple highlighter and pen. Now as you read, and as you write in your notepad, you get a new power. If something seems particularly important or interesting, just write with your purple pen or mark it with your purple highlighter. Then, magically (through my Wizard-of-Oz efforts), you’ll find that your memory system includes lots of prompts about that material, to ensure that you internalize it.
But at least when we started, the material which felt most salient to Alex wasn’t from a textbook or his own notes—it was from his tutoring sessions. Thankfully, he records every session with Otter, so he was able to send me audio with associated transcripts. What incredible material these are for a designer of a learning system! The conversational format externalizes much that would otherwise remain invisible to me, trapped inside Alex’s head.
Before I began this project, I was worried about how I’d get a clear picture of Alex’s evolving understanding. But through these transcripts, the tutors largely solve that problem for me. They ask questions and pose problems designed to interrogate Alex’s understanding. Through his responses, I receive a nuanced picture of confusion, confidence, frustration, interest, surprise, sluggishness, and facility. It’s richer material than I’ve gotten from any diaries, interviews, or observations I’ve done in the past. Part of the reason for that is that the tutor’s questions are about helping Alex, whereas when I’ve asked interviewees similar questions (“could you try to explain X for me?”) it’s about helping me, the researcher. There’s much more investment and connection in this context.
So one thing I’ve been doing is listening to these transcripts, and writing prompts to support anything important that comes up. The format makes “big a-ha moments” surprisingly clear. With fairly high accuracy, I can hear in Alex’s voice when he’s surprised, when he’s learning something new, when he finds something important. I mostly haven’t needed a purple marker cuing me to find the moments he’d want to internalize. But that doesn’t mean I can always tell what about the moment was surprising or exciting: in several cases, I identified the moment correctly but wrote prompts about the wrong aspect. And, of course, it’s time-consuming for me to find those moments through his tone of voice. We’d like to set up a sort of “clicker” that would let Alex mark, in real-time, important moments for me to review.
Tutoring sessions also create strong reference points to emotionally anchor the memory prompts. For example, in one case, Alex found himself confused in the middle of a difficult problem because he was mistaken about a fundamental property of matrix arithmetic. I wrote some abstract prompts to capture the relevant linear algebra, and he cleverly suggested: maybe I could include a “motivation” note with the prompts’ answers, to explain how the prompt connects to his confusion in the physics discussion he actually cared about. That seemed like a great idea. The questions I’d written were abstract and direct. It’s easy to imagine that a few months from now, one would come up, and he’d wonder: “why am I getting this random abstract math question about matrices? who cares?” I’ve certainly experienced that quite a lot in my own practice. But if I ground the question in a powerful discussion or experience or project, I suspect it wouldn’t be hard to reclaim my interest. Alex’s initial impression of the “motivation” notes has been quite positive. I’ll be curious to see how he feels in a few months.
One of my goals is to understand the connection between memory system prompts and practical fluency. In what circumstances does one support the other, and to what extent? Are certain kinds of prompts more important than others for that kind of transfer? In what ways do my current repertoire of memory practices fall short of producing practical fluency? I’ve gotten to see one interesting example of that last category in these tutoring sessions. Alex has lots of memory prompts about measurement of quantum systems, but there’s still a real sluggishness and hesitancy when he wants to actually write out the states of systems and manipulate them in the context of measurement. I think this may be in part because the memory prompts are all about laws and definitions and properties, but they don’t really practice applying that knowledge. I’ll be interested to experiment with some more “exercise”-oriented prompts.
The tutoring transcript format also suggests affordances which I might otherwise not have considered. For example, I noticed that sometimes when Alex brings up a question for discussion, the conversation ends up meandering onto another subject before the question seemed fully answered. I was able to mark these with comments like “Did this question get answered?” and “Are you still confused about this?” In some cases, Alex told me that he knew what was happening and decided it wasn’t important; in other cases, he found it useful to have pointed out that the confusion remained. He’d already established a queue of outstanding questions (partly, I imagine, as fodder for these tutoring sessions), so there’s a natural place to put questions which came up but remained unanswered. One can imagine a future learning environment surfacing these automatically.
One final note on these tutoring sessions. For Alex, the recordings were already quite transformative, long before I came along. Here’s a passionate ode to Otter, his audio transcription app, from one of our conversations: “Without Otter, I actually feel like I would be helpless. [laughter] … Reviewing the sessions… is like the core of being able to learn this stuff. … I just don’t retain enough on the first pass. [I also] don’t capture enough information to be able to make [memory system] cards with. I feel so held by Otter!” Properly humbling for me as a system designer.
Part of Alex’s comment there, by the way, is about the importance for him of reviewing his tutoring sessions. Before we started collaborating, he was already carefully going back through the transcripts, noting where he was still confused, and writing his own memory prompts. He expressed some initial suspicion of me writing the prompts for him: “Will that make me lazy?” Maybe. There really are trade-offs here. It’s complicated. Empirically, he’s been eager to use the prompts I’ve written for him. I’m hoping to understand some of these trade-offs better over time.
Talk-aloud review sessions
Another revelation for me came in the form of a fifteen-minute video clip. I wanted to understand how the prompts I was writing felt, a few weeks later. Where were they boring? Helpful? Off-target? Could Alex notice the way a single prompt had influenced his practical facility? So, at first, I asked for feedback, suggested he make some notes while he did his memory system reviews. These were somewhat helpful, but I wanted a lot more detail. I also worried those notes were a little too filtered, a little too detached. So I asked Alex if he’d be willing to use the iOS screen recorder to talk aloud while he did his review session.
And: absolute magic! I felt I was accessing a new level of insight about the practice of writing memory system prompts for someone else. Historically, I’ve gotten live per-prompt reactions from readers on their initial pass through a mnemonic essay. Those observations have been quite instructive, but they’re also limited—those readers don’t have enough distance on the prompts. From Alex, I get per-prompt reactions like:
- “I know the answer, but I’ve noticed that I don’t really understand why this is the answer. I’m just parroting.”
- “Whenever this one comes up, I find myself wanting to rush through it. Something about the wall of text.”
- “I’m answering this question by rote. I’m not really thinking.”
- “This feels like it’s basically the same as the question that came up a few prompts back.” (it wasn’t… but the fact that it feels that way suggests something interesting)
- “This one has felt really useful—I’ve noticed it’s come up a few times in tutoring.”
- “This was helpful at first, but now I feel like I really know it, and it’s annoying to continue being asked.”
- “I feel like this actually wants to be two questions.”
In some cases, this feedback led me to rewrite the prompts, or to write new prompts. In other cases, the right prescription was for some questions to get added to Alex’s outstanding question queue. In still others, the problems seemed more with the system itself. In any case, it seems clear to me that this format makes a much more effective feedback loop possible.
One common theme in Alex’s feedback is just how hard it is to really nail the target of the prompt. Often I’d written a prompt that used all the right key terms, so it seemed right initially—but when Alex practices it, he notices that it’s not reinforcing quite the right aspect. Or I’ve written a prompt about something that had confused him, and now he can answer it… but he’s still confused; there’s something important I failed to capture. All this rhymes with my experiences trying to get large language models to write good prompts. They’ll write prompt-shaped text, and it has all the right words, but most of the time it’s subtly (or not so subtly) off-target. Worse: it’s usually not at all straightforward to evaluate whether the prompt is on-target, nor to articulate the way in which it’s off-target. In many of these cases, I feel it may not be possible (for a person or a language model) to hit the right target on the first try. The prompt-writing process may truly need the feedback pressure from subsequent review sessions, to shape the prompts appropriately.
On a more mundane note, these recorded sessions seem like a good way to make low-hanging improvements to memory systems, by eliciting and working through a fine-grained friction log. For example: with a number of prompts, Alex noticed that he felt an impulse to move on without really thinking about them, because there was an imposing wall of text. This makes total sense in hindsight. He’s using Anki, and—I think because it was designed for vocabulary words—it has truly awful default typography for prose. More structurally, there really should be a hierarchical separation between the “answer” one is supposed to check, and a longer explanation one could read for more detail if one wants. When both are presented with the same appearance, the answer appears enormous. (We noticed the same problem in Quantum Country and put explanations behind a disclosable section on the backs of cards.) So I did a quick typography polish pass, and I styled extended explanation differently. I’ve included a before/after below; Alex reports that these prompts now feel much better.
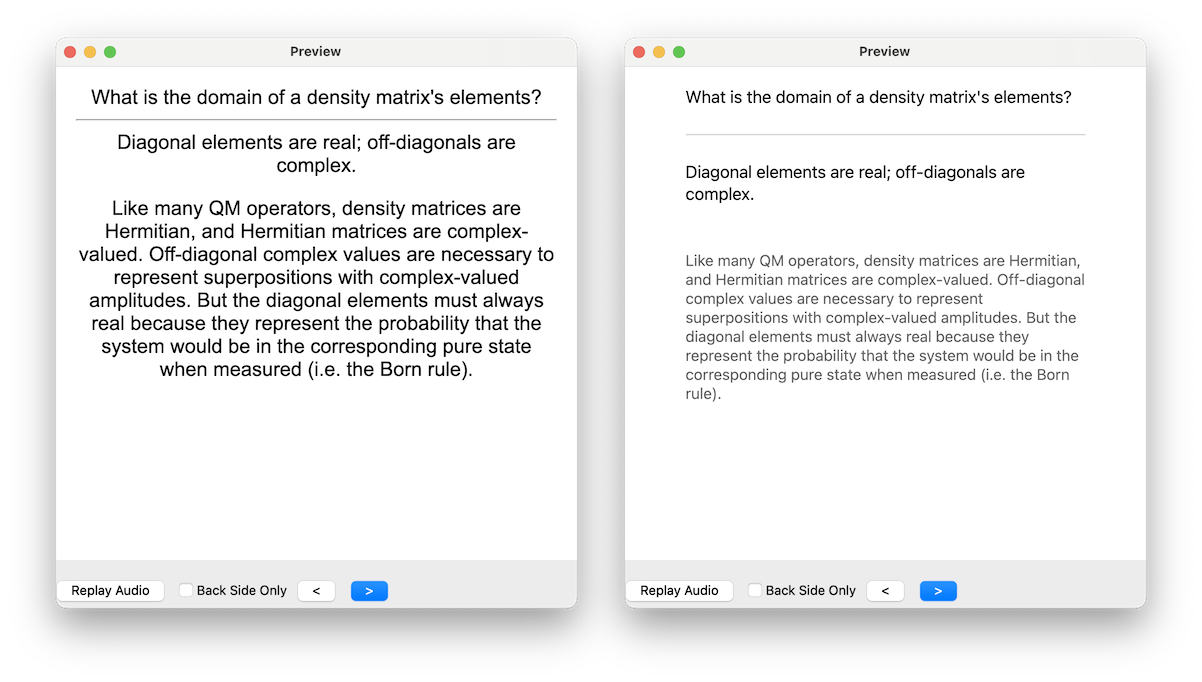
Stuff like that is easy. It’s not important, exactly. But a lot more iteration of that kind will help us see the actual boundary conditions of these systems much more clearly, without distortions from needless impediments.
Next
It’s only been a few weeks; I’m still getting my footing; we’re still figuring out the right way to work together. I certainly haven’t yet produced anything approaching a transformative augmentation. But I’m excited, I’m prototyping at a faster pace than I have in a long time, and I feel I’m learning a great deal, even if I can’t articulate much of that very concretely yet.
My immediate next challenge is to find a good way to “get inside” Alex’s learning loop. In our first couple rounds, he might articulate a question on Monday, have a tutoring session on Tuesday, get prompts about it from me on Wednesday, review them for the first time on Thursday, and get feedback in front of me by Friday. We’ve tightened that by a couple days, and daily conversations about his plans and barriers are helping me respond more quickly. Part of the trouble is that I’m building prototypes as I go, and of course that takes time. Maybe once my armory is a bit better established, it’ll feel easier to keep up.
One thing this experience is: intense. Intense, for both of us! Alex is trying to learn difficult material and contribute to an open question, on a very ambitious time frame. And I’m trying to make a transformative difference in his learning experience through second-party interventions. Both tasks feel awfully overwhelming. An air of commiseration helps the project, I think, but the strain is honestly quite palpable, alongside the constant sense of excitement. In any case, I’m looking forward to more.
My thanks first and foremost for Alex, for so vulnerably opening up his learning journey to me. I’d also like to thank Michael Nielsen, Gary Wolf, Robert Ochshorn, Ben Reinhardt, Joe Edelman, and Nick Barr for helpful discussions about this effort.
My work is made possible by a crowd-funded research grant from my Patreon community. If you find my work interesting, you can become a member to help make more of it happen. You’ll get more essays like this one, previews of prototypes, and events like seminars and unconferences.
Finally, a special thanks to my sponsor-level patrons as of January 2024: Adam Marblestone, Adam Wiggins, Andrew Sutherland, Andy Schriner, Ben Springwater, Bert Muthalaly, Boris Verbitsky, Calvin French-Owen, Dan Romero, David Wilkinson, fnnch, Heptabase, James Hill-Khurana, James Lindenbaum, Jesse Andrews, Kevin Lynagh, Kinnu, Lambda AI Hardware, Ludwig Petersson, Maksim Stepanenko, Matt Knox, Michael Slade, Mickey McManus, Mintter, Peter Hartree, Ross Boucher, Russel Simmons, Salem Al-MansooriSana Labs, Thomas Honeyman, Todor Markov, Tooz Wu, William Clausen, William Laitinen